Differences and usage of Optical Character Recognition, Robotic Process Automation & Accounting AI
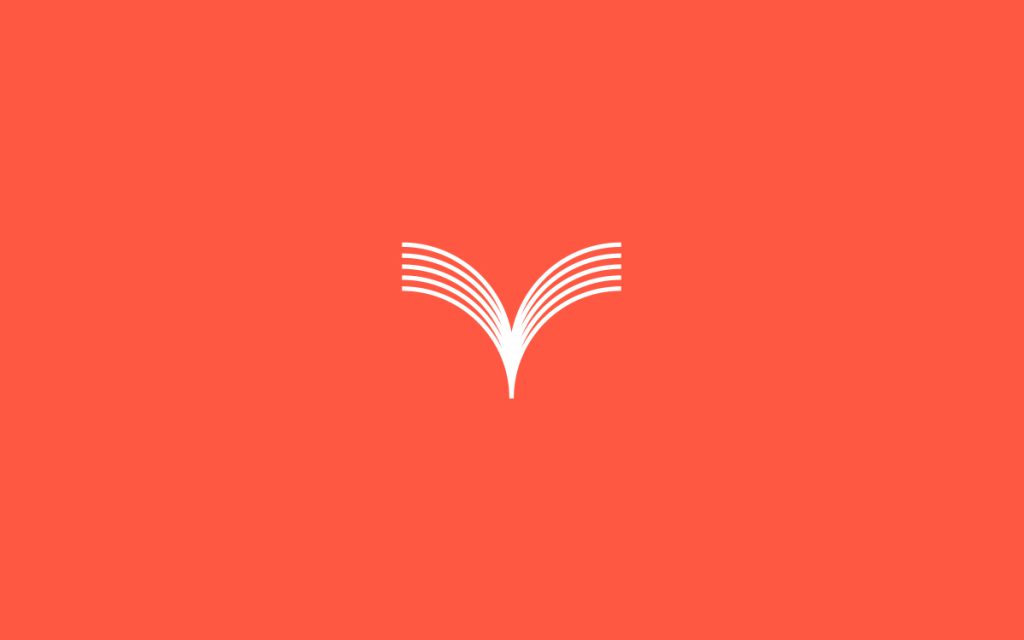
Are you engaged in the field of Accounts Payable? Then you are likely familiar with acronyms such as OCR (Optical Character Recognition), RPA (Robotic Process Automation), and AI (Artificial Intelligence) in the domain of Accounts Payable automation. However, gaining a comprehensive understanding of these terms, their operational mechanisms, their potential benefits, and the extent of automation they can introduce to your Accounts Payable team can be difficult. Let us initiate our exploration from the beginning of the process.
Data capture from PDF (or JPEG, TIFF,…) to AP/ERP software
If your interest lies in optimizing time efficiency within Accounts Payable by eliminating manual entry of invoice data, or the repetitive task of copying and pasting invoices from previous months with subsequent minor modifications, you may already be informed with the concept of OCR. How does OCR precisely work in the context of Accounts Payable?
OCR, an acronym for “Optical Character Recognition,” essentially involves the conversion of text, whether handwritten or printed on invoice images (e.g. PDF, TIFF, JPEG, or other image formats), into machine-encoded text that is both searchable and editable (e.g. XML, JSON, CSV, etc.).
Through the utilization of OCR, the manual input of invoices becomes obsolete. Instead, OCR software can extract the invoice data—such as invoice type, invoice number, supplier and buyer information, reference numbers, dates, amounts, VAT ratings, and even line items with corresponding descriptions. This facilitates accurate invoice booking in accordance with booking policies.
Contemporary OCR solutions are often enhanced by Artificial Intelligence (AI) and Machine Learning (ML) engines. These advanced technologies contribute to the more effective recognition of diverse invoice templates and the extraction of invoice data with heightened precision. In simpler terms, when a new invoice is received, marking the relevant data in the new template enables the OCR solution, with a high probability, to automatically extract the required information from subsequent invoices based on the template processed previously.
While numerous OCR solutions offer automation capabilities for posting data, it is important to note that, in most instances, this involves a “copy & paste of the last selected values”, or just easy algorithm of used values feature rather than a true AI engine for predictive posting in the background. The implications of erroneous invoice bookings resulting from human error underscore the ineffectiveness and inappropriateness of such approaches. Fortunately, improving this situation is a straightforward process. How? Let´s continue!
Rule-based automation
RPA, an acronym denoting “Robotic Process Automation,” also referred to as Software Robotics, is instrumental in automating repetitive tasks. Within the context of Accounts Payable and invoice booking, RPA solutions can facilitate the pre-filling of posting fields (e.g. General Ledger, VAT Code, Cost Center, Project, etc.) or even entire workflows, leveraging criteria such as Business ID, Reference Number, Item Description, and other similar parameters.
Additionally, within the realm of rule-based automation, notable features include “Purchase Order Matching” (referred to as “PO Matching”) and “Contract Matching”. These automation processes are rule-based as they require specific information to trigger the corresponding rules within the Accounts Payable/ERP system. PO Matching involves automation based on data such as the Purchase Order Number, initiating the matching of an invoice to a pre-approved purchase order. Conversely, Contract Matching comes into play when an invoice includes a Contract Number, prompting the automation (matching) of the invoice to the original vendor contract.
Despite the persistent efforts of many companies over the past decades to achieve 100% Purchase Order (PO) automation, a considerable number have fallen short, reaching only 30% or 40%. Even when PO matching is implemented, key questions must be addressed before making any decisions:
- Are all PO invoices consistently matched 100% to their corresponding Purchase Orders?
- Do the purchase orders include all the required dimensions that need to be extracted from the PO and filled in on the incoming invoice, following your organization’s booking policy?
- Can the AP team easily process an invoice if the matching has not been triggered?
- And what is the procedure when PO matching fails to activate? Consideration should be given to the time spent on communication between the business units (colleagues entering the approval process) and the Accounts Payable team.
If any of these questions yield a “NO,” there is undoubtedly room to enhance the current level of automation.
AI as a Booster or a Leader of current automation
AI, acronym for “Artificial Intelligence”, simulates a human intelligence process by computer systems. Nowadays, we can see many different AI tools within our daily lives – for instance, the virtual agent we talk to before connecting with the real person from the bank department we need to talk with, or a chatbot when we want to report any technical problem to the internet provider.
What a wonderful news – AI has finally arrived also in the domain of Accounts Payable.
Snowfox.AI predicts data that does not exist in the invoice, but is an integral part of the invoice processing – selecting the posting data such as General Ledger Account, VAT Code, Cost Center, Project, WBS Element, etc. In addition to the booking dimensions Snowfox.AI is also capable of providing you with an approver/reviewer of the invoice as well as the whole approval area if needed.
Are you interested in how AI can process your organization´s incoming invoices?
No problem, we can do an AI capability test for you. The process involves initiating AI with a batch of historical data, comprising invoice images (in formats such as PDF/TIFF/JPEG), structured invoice data (invoice metadata post OCR data extraction, in formats such as CSV/XML/JSON), and postings & routing information from the AP or ERP system (CSV/XLSX/XML/JSON). These data sets can be easily exported from the current AP/ERP software or obtained from the system’s provider.
Approximately 70% of historical data is utilized for AI training, resulting in a specific AI model tailored to each company’s unique requirements. The remaining 30% of historical data is treated as “new invoices,” and AI predictions are compared with existing data, showcasing how AI can predict invoices in a visually intuitive manner.
In addition to the questions about how Snowfox.AI works and what the AI capabilities are, we often get the following questions:
- How does Snowfox.AI work in real production when I already have rule-based automation?
- What happens when an accountant makes a mistake?
- What if we receive a totally new invoice?
Answers are simpler than you thought. AI seamlessly cooperates with existing rule-based automation. In real production, AI predicts only those invoices that cannot be processed by the existing rules. In situations where creating and maintaining rules is challenging, AI can efficiently handle all incoming invoices. If an accountant made a mistake, AI will not automatically predict the last selected value. AI awaits some time to take into consideration this change and updates the posting logic.
Additionally, if changes are made to the chart of general ledgers or other dimensions, the AI model can be easily re-trained. When faced with a completely new invoice, AI leverages historical data to make predictions based on past purchases within all historic invoices.
Is achieving “touchless process” even possible?
The answer is a resounding YES. AI in cooperation with AP/ERP system has the capability to directly transfer predicted invoices into the bookkeeping software without requiring any approval process. This streamlined approach allows significant time savings by enabling the identification of invoices that AI can autonomously handle. How simple is it, right?
Whether your current rule-based automation falls short of expectations, or you are keen to explore how AI can rapidly enhance your automation processes, we invite you to download our guide and schedule a discussion with us to delve further into the capabilities of Snowfox.AI.